Computer vision and natural language processing are closely related to daily life. Referring expressions, such as "please give me the white book on the desk," are common in people's social conversations and professional interactions. Reference expression segmentation focus on the relationship between subjects in an image, which is a combinational research are of the vision and language. Referring expression segmentation is an image segmentation algorithm based on a sentence that aims to segment the object mask referred by the expression.
A general solution to this task is conducting the multi-modality feature by concatenation of the image, expression and spatial coordinate features directly, which is in a coarse fusion method. Some noise may be introduced, which may affect the understanding of multi-modality content. These methods do not consider the relationship between vision and language for multi-modality feature fusion.
项目组人员:
赵重阳 2017级计算机科学与技术专业;
南京 2018级计算机科学与技术专业;
张宜千 2018级计算机科学与技术专业
项目指导教师:从润民
推荐级别:北京市级
研究背景:
计算机视觉和自然语言处理与人们的日常生活息息相关。自然语言表达式经常出现在人们的社交对话和一些更加具体互动中,例如,“请给我课桌上的白色书”。有参考图像分割是通过结合计算机视觉和自然语言处理中的文本表达式来描述图像中多个对象之间的关系。有参考图像分割是指根据自然语言的描述找到目标区域,这是一种基于自然语言的描述进行图像分割的算法。本课题主要研究有参考图像分割任务,着重探索跨模态信息融合与交互的机制。
应用场景:
我们假设了两个有参考图像分割的应用场景:
1) 智能人机交互:根据输入任意的语音或文字,对复杂场景图片进行智能抠图。
2) 对于不会使用PS软件的用户,也可以完成简单的图片编辑工作,如,证件照换背景。
项目创新点:
1) 提出了一种语言调制融合 (L2iMF) 模块,该模块首先利用局部和全局的语言特征对视觉特征进行调制,感知所有可能的实体,然后构建跨模态实例关系图来定义关系,以突出目标物体,同时抑制其他不相关的物体。
2) 提出了一个渐进式的实例交互融合 (PI2F) 模块,将单词分成4类, 包括物体、属性、关系和无关单词。在这些类别的指导下, 通过交互式的方式逐步缩小目标集合的范围,最终获得融合的跨模态特征。
3) 提出了一种自适应的多尺度融合模块,以整合高级和低级特征信息,获得一个对不同模态有着综合理解的特征。
4) 大量实验证明了我们提出的模型相较于现在其他先进的算法达到了极具竞争力的性能。
项目成果:
1. 提出的算法模型性能达到了世界先进水平。
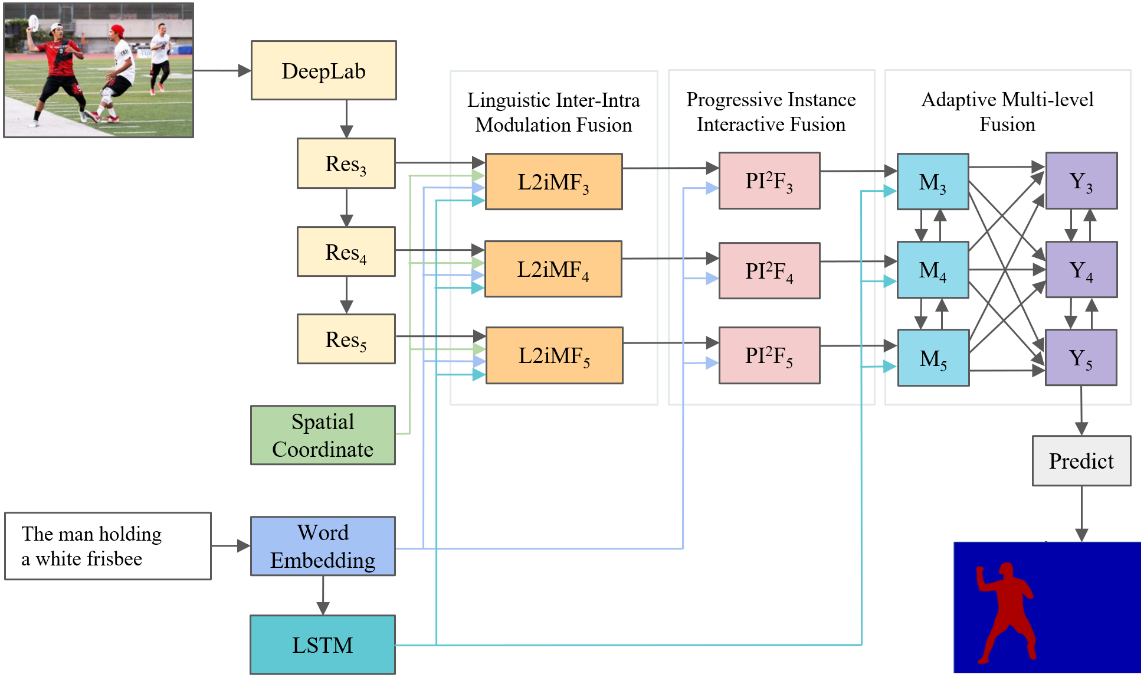
图1:算法模型示意图
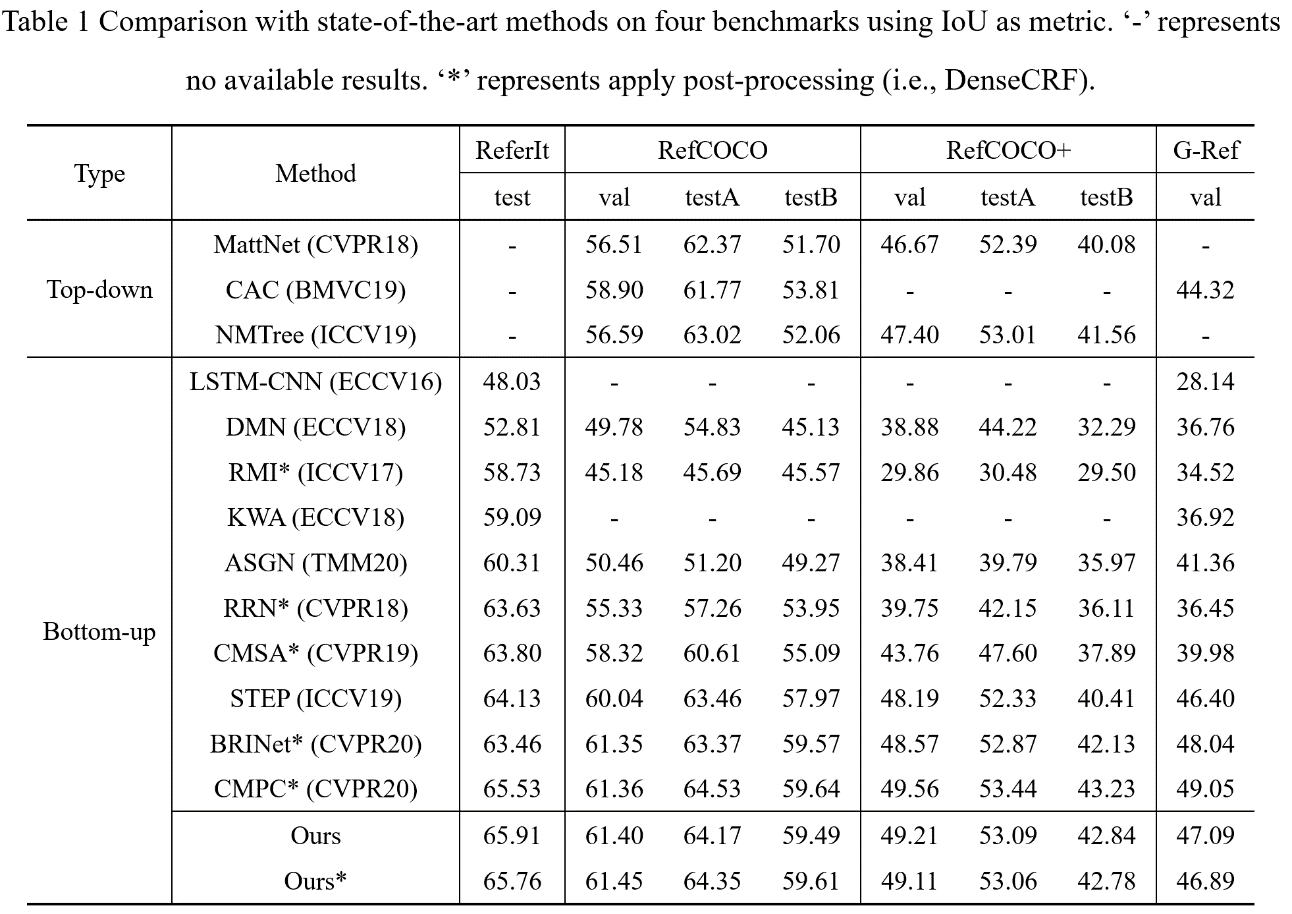
图2:模型性能对比
2. 开发了业内首款面向人机交互的智能AI抠图软件。
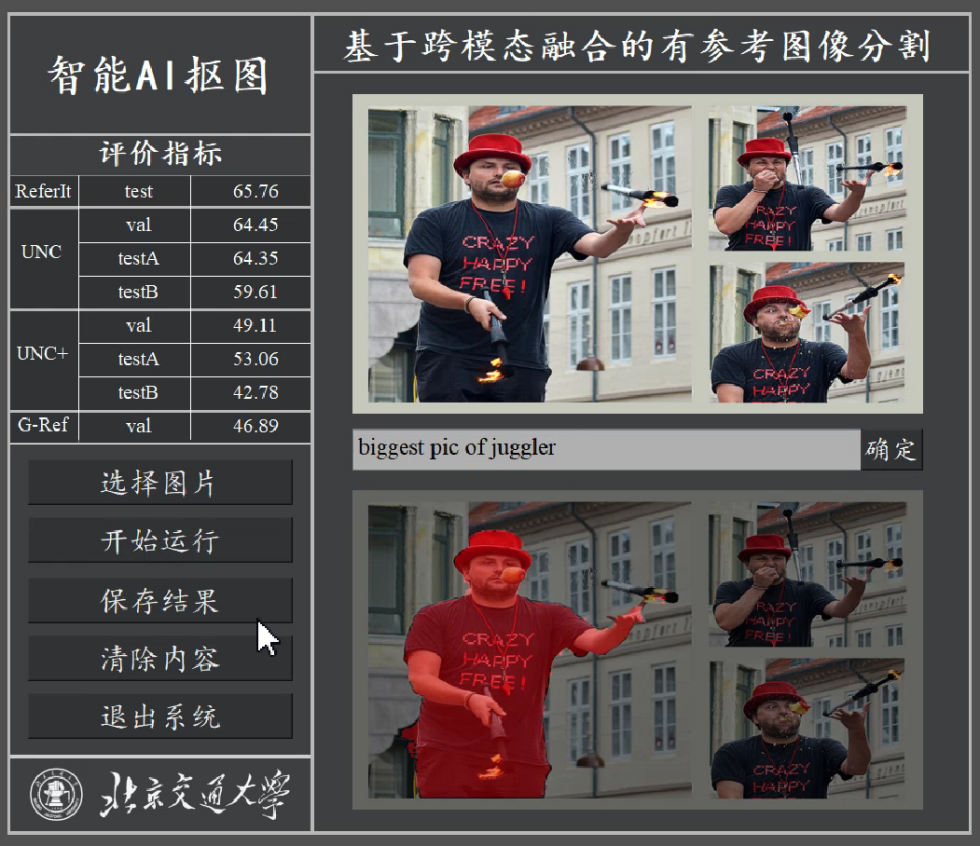
图3:面向人机交互的智能AI抠图